RETAIL
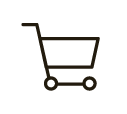
Personalization at Scale
+64%
Improvement in overall ROAS
+35%
Discovered Audiences
10,000%
Facebook Messenger ROAS
Big box retailer seeks efficient personalization & discovers a partner in growth along the way
“During Peak, we used to manually email product feed updates every half hour in addition to a daily ‘out of stock’ update. That meant employees had to be on call at all times to make manual updates, which created room for error and took a toll on personnel at that time of year. The Albert tech team automated these email updates for us – extending well beyond the initial scope of our test. They are a nimble, savvy partner.” - SVP Ecommerce
About
A big box retailer with a massive assortment of products and many different shopper types faced a familiar challenge: how could personalization in paid social be achieved efficiently? Finding and running every possible combination to reach their diverse audience felt like a near-impossible task, bound to be labor, time and cost-intensive – with messaging and product complexities that needed to be unraveled in order to achieve true audience relevance. They realized they needed a partner able to take on and simplify the challenge of efficient personalization across audiences, creatives and tactics to drive meaningful results.
Challenges
The first step was to design a split test, positioning Albert against their current method of paid social advertising. Like many large advertisers, this retailer had several matched geo tests running concurrently, meaning that split testing by geography would likely contaminate learnings. The Albert team found an imaginative hack. Using Facebook’s Brand Lift Study methodology, (which divides up the entire audience into segments that are equally matched in terms of behaviors, demos, etc.) marketers are able to hold back a percentage of audience as control group that is not advertised to, while the rest is exposed. By using these control and exposed segments as A & B test groups, the Albert team and client were able to split the audience to create mutually exclusive groups to test against.
Solutions
Once the challenge of separate, matched audiences was addressed and the campaign was launched, Albert immediately began the optimization process. From the start, Albert was at parity or slightly better than previous methods. As the autonomous AI learned, results steadily improved. Ultimately, Albert was able to achieve outcomes 64% better than control methods at a consistent rate over the course of the campaign, which ran through Black Friday and into the holiday period, Albert’s performance trend was significantly more stable than the human-operated campaign. As the results unfolded, marketers watched in Albert’s 24 x 7 dashboard which had been customized to display their bespoke business metrics.
Results
The seasoned mass merchant marketing team learned that deploying an intelligent machine to run paid social marketing will not only deliver consistent and improved results over control campaign management in peak seasons, but that there are some things only an autonomous, self-learning AI can uncover. For example, Unlike the human managed campaign that targeted women 25-54, Albert targeted everyone, looking for valuable opportunities. For this mass merchant retailer, 65% of the budget ended up being spent against their core women 25-54 audience. But Albert found that the remaining 35% was better spent against men, 20 somethings and 60-somethings to draw in incremental sales. Part of the 64% improvement in results that Albert drove is a reflection of reducing spend on known audiences and finding opportunities outside them, at an optimal threshold. Tactics also varied from the tried and true. Albert found ways to make Collection and Messenger ads work. On Black Friday, Albert autonomously made a $3,000 investment in Messenger that yielded almost $1mm in revenue because it was such an efficient placement. The AI had noticed that while Messenger had a small audience, it was a consistent top performer. Because Messenger would never be the biggest spend or revenue driver, a human campaign manager would not know for certain how to execute a profitable test as it would be so easy to overspend and kill results. Albert was able to deduce that with exception of Black Friday and a few key weeks, a spend of just around $1,000 a week made the most sense – primarily because it had a consistent ROAS of 10,000%!